Decarbonising the Shipping Industry using Machine Learning
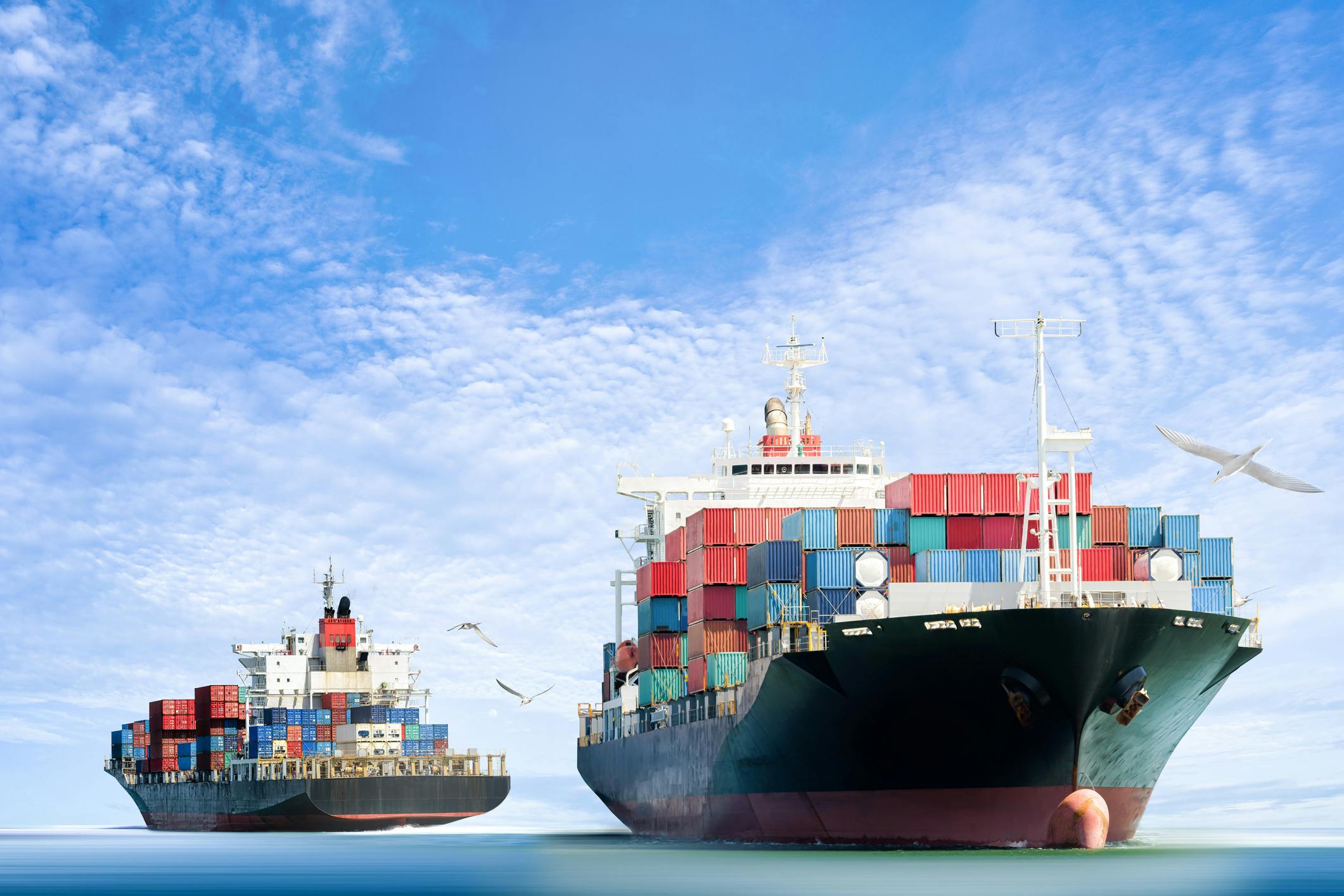
Oliver Gindele, Chief Innovation Officer of Datatonic, outlines five recommendations on how Data & Machine Learning can reduce carbon emissions in the shipping industry. He argues that while improvements in infrastructure and regulation changes can take years to implement, the industry can use today’s data technologies to tackle one immediate area first: Operational Efficiency.
The path to a decarbonised future in maritime transport
The impact of greenhouse gas (GHG) emissions on accelerating the global climate crisis has been made clear in recent years. Luckily, determination to reduce emissions and to decarbonise the worst offending industries is increasing worldwide.
One particularly important sector is the shipping industry, which alone is responsible for around 2.7% of global CO₂ emissions(1). Shipping also accounts for about 80% of the volume of global trade(2).
To tackle the climate crisis and to achieve the goals of the Paris Agreement, whole industries, not just individual corporations, need to cut their carbon emissions and do so fast.
The International Maritime Organization has announced ambitions to halve international shipping GHG emissions by 2050 and reduceCO₂ emissions by at least 40% by 2030. (Source: Shell, 2020)
Yet, the path to a decarbonised future in maritime transport is still filled with challenges.
Sector leaders point to the following concerns:
- Lack of regulatory incentives, such as tax cuts or carbon levies
- Lack of technological alignment between carriers, countries, ports and manufactures.
- Time & upfront costs to modernise vessels and infrastructure
- Time & upfront costs to improve infrastructure and available technologies
Despite these listed challenges, there are plenty of opportunities to act now.
By levering the right types of data, fuel savings in the range of 5-20% can be achieved, leading to huge reductions in emissions. (Source: GreenSteam, 2019)
Datatonic sees Machine Learning and Advanced Analytics as driving technologies to decarbonise shipping in the near term. Modern data technologies can target an immediate area in the fight to mitigate climate change: Operational efficiency.
Here are my five recommendations using Data & Machine Learning:
1. Optimising shipping routes
Global shipping routes offer massive potential for improving operational efficiency. An estimate by a shipping operator states that a 10% CO₂ reduction can be achieved by optimising arrival times like airlines.
Historic data on shipping routes, timetables and delays is often available to shipping companies and machine learning algorithms for route planning have matured over the past decades. Leveraging this data in order to spot inefficiencies of their networks and to automatically suggest improved routes on-demand can be a quick way for many shipping operators to reduce their emissions.
Another approach to improve shipping routes and minimise travel is matching the consumer demand as closely as possible to avoid unnecessary transport. 2020 has shown how a global pandemic can massively disrupt supply chains, creating vast imbalances between supply and demand. Applying accurate forecasting to estimate future demand for each region can help shipping companies to right-size their transport modes.
Here, the state of the art demand forecasting models that the retail sector developed in the past decade can be straightforwardly reapplied for improvements in short and long term demand sensing.
2. Fleet modernisation
Container ships take years to build, require a lot of capital and have many decade-long lifespans. The risk of betting on the wrong emerging fuel technology is substantial. This means that in reality, operators have to manage a changing yet diverse fleet composed of traditional HFO fuelled, electrical alternative fuel vessels such as hydrogen powder ones.
A data-driven approach can help with managing such a complex portfolio of vessels and fuels to ensure cost-effective operations and fuel availability. Here, forecasting of fuel prices at different bunkering sites can be employed together with machine-learning-driven optimisation algorithms.
This will allow operators to make short term changes in their routing to adapt to fluctuations in the supply of alternative fuels. They can also make long term strategic purchasing decisions on how to modernise their fleet.
3. Predict vessel fuel consumption
Digital fuel meters are not commonly installed in today’s fleets. However, modern vessels do collect large quantities of engine and performance data, such as speed, position and engine parameters. It has been shown (see here and here) that these data points can be used to gauge real-time fuel consumption of vessels through predictive machine learning algorithms.
These predictions can be further merged with additional data sources such as AIS vessel position data. AIS data tracks all marine traffic and can deliver insights into previous or alike journeys of similar vessels to make predictions of fuel usage even more accurate. Insights generated by analysing these real-time data points and machine learning predictions can help crews to reduce fuel wastage and thereby cut emissions.
4. Predict vessel emissions
In order to reach the goals of reducing GHG emissions accurate knowledge of where these emissions originate from is crucial. Machine learning approaches have been proven to estimate different types of emission based on available vessel data and dynamic engine parameters (see here and here). Based on these machine learning predictions, operators can assess in real-time where emissions emerge and derive insights into the carbon impact of their whole operations.
5. Forecast weather and delays
Machine learning has demonstrated great promise in highly local weather forecasting (see here). Modern fleets of vessels equipped with IoT sensors, together with traditional weather data sources can deliver unprecedented accuracy in predicting the weather and ocean conditions along the world’s shipping routes.
Applying deep neural networks onto that data for short term forecasting can help improve routes to avoid perilous weather scenarios that will slow down journeys, increase fuel consumption and add substantial risk to the crew.
Furthermore, accurate machine-learning-driven forecasts of weather and ocean currents can improve estimates of arrival times and spot potential upcoming delays, adding further opportunity for improving operational efficiency.
Machine Learning can drive impact within weeks, not months
Although the time horizon over which the International Maritime Organization wants to reduce their carbon footprint appears to be long, the time to take action is now.
Fortunately, the machine learning approaches lend themselves to prove value very quickly. If suitable data sources are available these methods can demonstrate impact in weeks, and do not rely on heavy upfront investment or infrastructure changes.
Breakthrough innovation starts with experimentation and the AI technologies are ready to decarbonise shipping. When it comes to unlocking impact from these approaches it is up to the industry players to move from the lab to operational environments faster than ever.
(1) https://www.shell.com/energy-and-innovation/the-energy-future/decarbonising-shipping/
(2) https://www.shell.com/energy-and-innovation/the-energy-future/decarbonising-shipping/