Prompt Engineering 101: Using GenAI Effectively
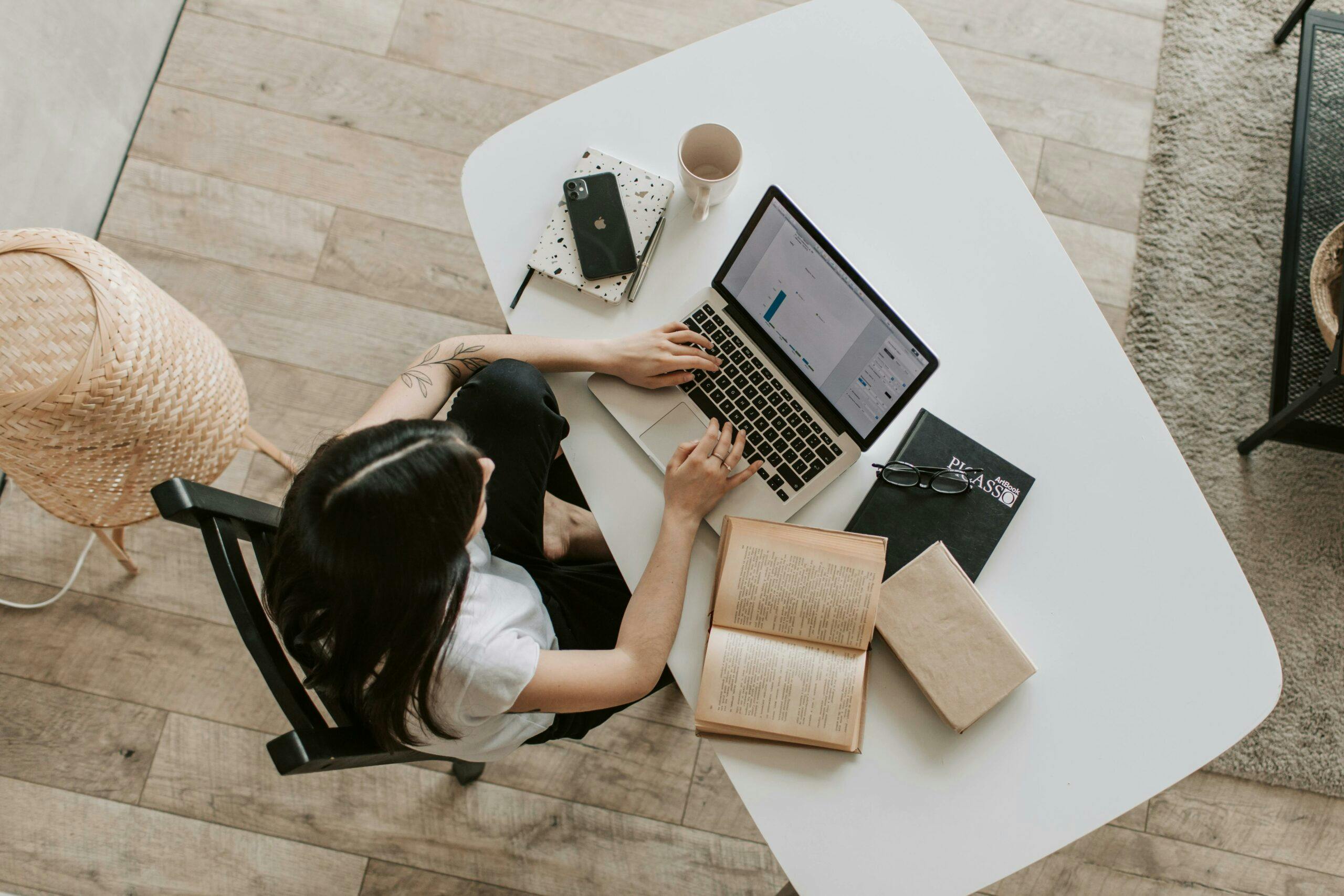
Author: Thomas Udale, Senior ML Trainer
Prompt engineering has quickly become a hot topic in the world of Large Language Models (LLMs) for a good reason; without it, the models are much less capable of generating relevant outputs. Prompt engineering gives users and enterprises the tools to tune both the knowledge base of the model and the output it produces. In this blog, we explore the relevance of prompt engineering and look at how consumers and enterprise users can improve LLM output to create more value with Generative AI.
What is prompt engineering?
Prompt engineering is “the practice of designing inputs for generative AI tools that will produce optimal outputs”. This allows businesses to provide the LLM with additional information and context, that improves the quality and relevance of its responses to questions or tasks.
Traditional (if two years is long enough for traditions) prompt engineering focused on changing the user prompt to change the content, style, and format of model responses. Adding in context, asking for bullet points, or even adding a few examples of prompt-response pairs are all ways in which we can change the model output.
These are time-efficient methods for improving the quality of response by bringing output closer to our implicit expectations. We can even use prompts to guide the model into better logical reasoning by explaining the required thinking process, through Chain-of-Thought (CoT) prompting.
Why do we need prompt engineering?
The key idea in traditional prompt engineering is to better leverage the internal knowledge in the models, gleaned from billions of training sentences. The models have internalized a great number of patterns from this training data, including any biases, false information, and inappropriate language! Guiding the model to replicate patterns closer to our desired output is the end goal of traditional prompt engineering.
Training LLMs is difficult. It required vast quantities of data, data centers full of GPUs and TPUs whirring away for days on end, as well as strong technical knowledge requirements around networking to move data and coordinate hardware for training.
This is why larger companies, such as Google have produced many of the top-performing models of the last few years. This also means that they have control over the data that is fed into the models.
The history of LLMs and prompt engineering
Looking at the context window of LLM models helps us to understand why prompt engineering is now so relevant. In 2017, an early LLM, BERT, had a context window of 512 tokens. That meant you could feed roughly 500 words into your model at once. This is a big limitation that would be overcome as models became ever larger.
The largest models now have context windows of over 1 million tokens, which means you can feed novels into them. This has also opened the door to bringing in external knowledge to an LLM via the prompt.
The impact of prompt engineering
Techniques such as retrieval augmented generation (RAG) allow models to bring in relevant information from external sources into the prompt, where the model can be asked to make sense of and use the new text.
Without having to retrain the model (a time-consuming, expensive process), enterprises can leverage their own unstructured data to improve LLM output. This is the real step forward in prompt engineering. Expanding the set of patterns the model has access to, with information particularly relevant to your task is a vital way to bring enterprise use cases, such as chatbots, knowledge workers, and analytics assistants, to life.
Conclusion
To summarise, prompt engineering broadly falls into two categories. Firstly, to make better use of the internalized patterns from the training data. Second, to help bring in external knowledge from company documentation, enhancing the patterns that the model can use to generate responses. With prompt engineering, users can receive more relevant and useful outputs from LLMs, increasing efficiency and giving them the information they need faster.
Want to get started? Secure your spot on our free Prompt Like a Pro taster session and embark on a transformative journey into the world of Generative AI. Visit our event page for more information and to register.
Turn Generative AI hype into business value with our workshops and packages. Get in touch to discuss your Generative AI, ML, or MLOps requirements!