Reducing Emissions and Operating Costs for 5G Providers
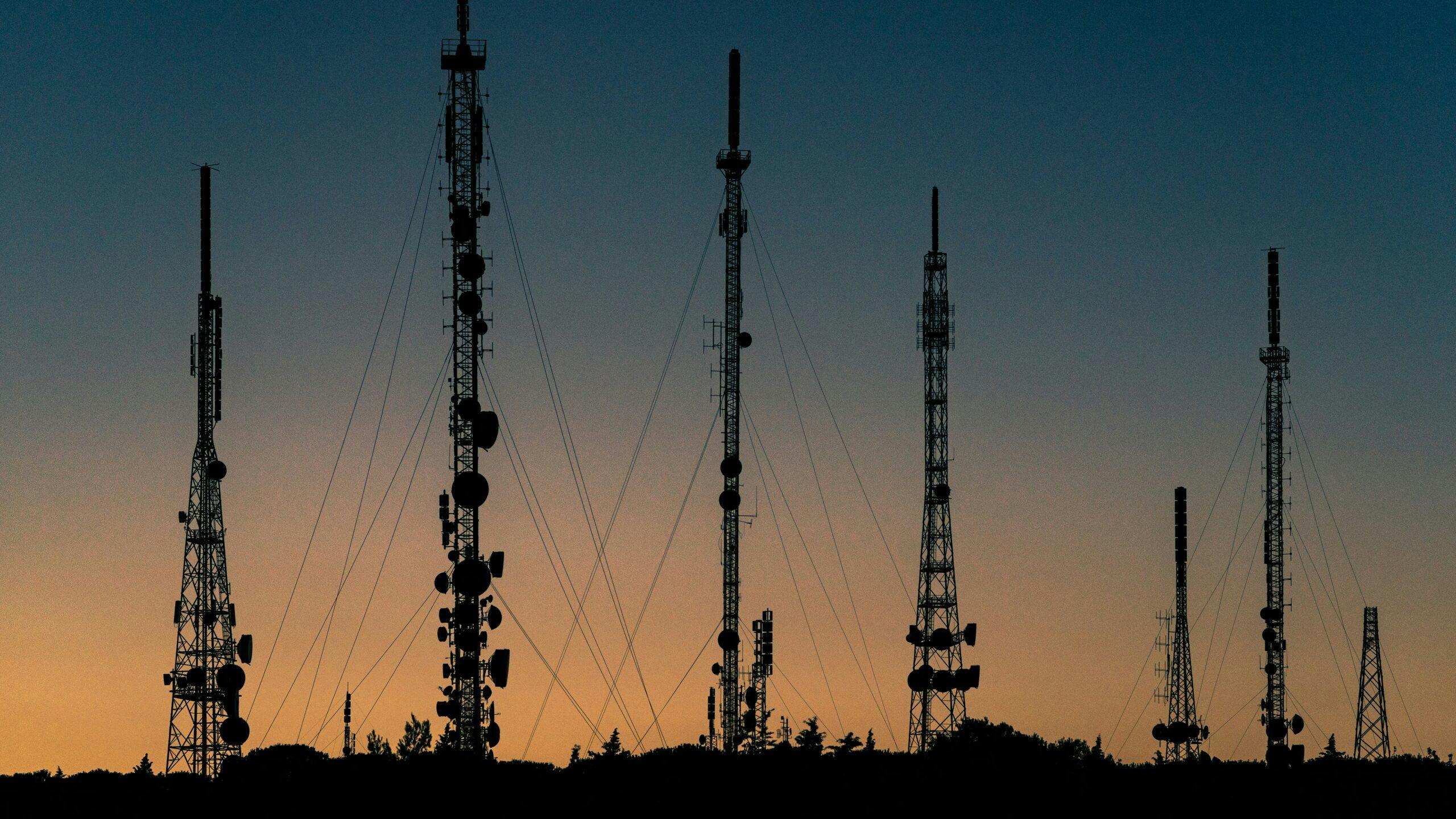
Authors: Fenella Slingsby-Smith, Business Analyst and Olivier Philippe, Strategy Specialist
In 2022, the global 5G market was $107 billion and it’s expected to more than triple in 5 years to $331 billion by 2027. 5G is much faster in unlocking benefits for customer experience and new revenue for providers.
However, there are a few challenges for providers to consider before they can unlock this business value. One of the main costs introduced by 5G networks is the increase in energy consumption, and therefore costs. As a result, these businesses need to strike a balance between over-provisioning leading to excess energy consumption and under-provisioning leading to poor customer experience and therefore client churn.
In this blog, we’ll look at a potential solution to this: using Reinforcement Learning to optimise network energy efficiency, without compromising service to the customers, to control the optimal set of activities for each station based on the information available at each point in time.
Introduction
Mobile networks have significantly evolved since their inception, starting with just voice communication and growing into sophisticated data networks that power our interconnected world. The latest generation, 5G, holds immense promise for providers and customers.
In this blog, we explore the following:
- The demand for 5G
- Mobile Network History – 1G to 5G
- Current Issues with 5G Adoption
- The Synergy of 5G and AI
- Energy efficacy solution
- Technical deep dive of solution
The Demand for 5G
For providers, 5G represents a tremendous opportunity to meet the ever-increasing demand for data-driven services. The new network enables significantly higher data transfer speeds – up to 10 times faster than 4G, lower latency, and increased network capacity. This makes it possible to deliver seamless streaming experiences, support the Internet of Things (IoT), and enable groundbreaking innovations like autonomous vehicles and remote surgery.
Customers and businesses also stand to benefit greatly from 5G. Enhanced mobile broadband speeds and low latency will revolutionise various industries, such as healthcare, manufacturing, transportation, and entertainment. From real-time remote monitoring of patients to precision control of industrial processes, 5G will unlock a world of possibilities, enabling faster and more efficient operations.
Mobile Network History – 1G to 5G
The history of mobile networks can be traced back to the early 1980s when the first generation (1G) was introduced, enabling wireless voice communication. This was followed by 2G, which brought in digital technology, allowing for text messaging. With the advent of 3G, mobile networks took a significant leap forward by enabling faster data transmission and basic internet connectivity.
4G, the previous generation, brought about substantial improvements in speed, capacity, and latency, paving the way for advanced applications and services. And now, we stand at the cusp of the fifth generation (5G), which promises unprecedented capabilities and transformative potential.
Figure 1: The increase in innovation of networks over time.
Current Issues with 5G Adoption
While the anticipation for 5G is high, there are some challenges to its adoption:
Energy consumption: The adoption of 5G by telecommunications companies brings significant challenges in terms of energy consumption. The higher data transfer speeds, increased network capacity, and denser infrastructure requirements of 5G networks result in a surge in energy usage compared to previous generations.
Telecommunication companies also need to deploy additional base stations and network equipment to support the extensive coverage and connectivity demands of 5G. These infrastructure expansions further contribute to elevated power consumption levels. Additionally, the advanced technologies and functionalities of 5G, such as massive MIMO (Multiple-Input Multiple-Output) and beamforming (a particular processing technique for signals that allow for directional transmission or reception), require continuous power supply, exacerbating energy demands.
The substantial energy consumption not only impacts the operational costs of telecommunication companies but also raises environmental concerns due to increased carbon emissions.
Cost of Implementation: Implementing 5G networks requires substantial investments by telecommunication providers. Upgrading existing infrastructure, installing new base stations, and deploying advanced equipment can be capital-intensive. The cost of spectrum licenses, technology acquisition, and network optimisation further add to the financial challenge.
Infrastructure and Deployment Challenges + Delays: The rollout of 5G networks involves substantial infrastructure upgrades and the deployment of new equipment. This requires collaboration between telecommunication companies, government authorities, and regulatory bodies.
The Synergy of 5G and AI
One of the key enablers for the success of 5G networks is the integration of artificial intelligence (AI). AI brings intelligent automation, predictive analytics, and enhanced network management capabilities to the telecommunications industry. It enables dynamic network optimisation, efficient resource allocation, and proactive issue resolution.
AI-driven solutions empower telecommunication to gain valuable insights from the massive amounts of data generated by 5G networks. This data can be utilised to optimise network coverage, identify bottlenecks, and personalise services based on customer preferences.
Energy Efficiency Solution
The transition to 5G requires denser infrastructure deployment, resulting in increased radius coverage overlap compared to previous generations. This shift places a heightened focus on the management and coordination of network infrastructure to achieve optimal performance and scalability of coverage. A large part of the infrastructure needed is the base stations.
There are three types of base stations, macro, micro and pico. The term macrocell (macro) is used to describe the widest range of cell sizes. Macrocells are found in rural areas or along highways. Over a smaller cell area, a microcell (micro) is used in a densely populated urban area. Picocells are used for areas smaller than microcells, such as a large office, a shopping centre, or a train station.
Often, telecommunication companies either over-provision their network resources leading to inefficiencies or under-provision them, which can impact customer experience. Management must navigate the tradeoff between network performance and energy efficiency; this often leads to over-provisioning given the focus on customer experience, leading to excessive energy consumption throughout the industry. Notably, base stations alone account for a staggering 57% of energy consumption across the industry.
Machine Learning can be used to manage cost and environmental impact whilst maintaining high-quality service to users. This can be achieved by creating a Machine Learning model to adjust the number of active base stations depending on traffic and users’ needs.
Energy Efficiency: Technical Deep Dive
Machine Learning can optimise which pico base stations should be active or in sleep-modus at any given time; the increasing complexity and scale require an automated solution to optimise energy consumption. Incorporating ML into this automated solution can save significant energy costs, whilst retaining great service to users (see Figure 2).
Figure 2: On the left, we see the current infrastructure set-up leading to excessive energy consumption as all base stations are on. On the right we see the ML-optimised base station giving the same coverage to users whilst limiting energy consumption.
Reinforcement Learning is the state-of-the-art training method that can learn to make optimal decisions in a highly adaptable, dynamic environment. You can find out more about how this works here. We can use Reinforcement Learning to train an agent to learn the optimal set of active base stations based on the information available to the agent at a certain point in time, which we call the state.
Let’s dive a little bit deeper into the key components of the solution:
1. State: The state is defined as a set of locations of User Equipments (UEs) within a pre-defined user grid (brown area in Figure 3) in the DeepMIMO situation.
Figure 3: (left) The methodology is inspired by the paper “Increasing Energy Efficiency of Massive-MIMO Network via Base Stations Switching using Reinforcement Learning and Radio Environment Maps” by M. Hoffman, P. Kryszkiewicz and A. Kliks.
(right) A public dataset, DeepMIMO Scenario 01 (Outdoor 1) was adapted to obtain a similar scenario as in the increasing energy efficiency paper [1]. This is done by reducing the number of active base stations and the range of locations the User Equipments (UE) can find themselves in.
2. Actions: The actions are a binary vector representing the status of the base stations. In total, 6 base stations are considered where one macro and 5 pico base stations. A resulting binary vector for action 0, looks like 0: (0, 0, 0, 0, 0, 1), representing the 5 pico base stations to be in the sleeping modus and the macro station to be active. All base stations to be active correspond with action 31, represented as: 31: (1, 1, 1, 1, 1, 1).
3. Rewards: The reward depends on the energy efficiency and the number of served UEs. To compute the energy efficiency is computed based on the power consumption and the average UE bitrates.
4. Agent: The agent is the decision-making algorithm trying to optimise the energy efficiency, without compromising the number of served UEs. The agent will choose an action based on the current state. This action corresponds with a certain configuration of the base station’s modus. If the agent makes a good decision, it will get rewarded, if not, it will get penalised.
5. Environment: The environment is a Heterogeneous network with 5 pico and one macro base station. As we don’t have real data available a simulated environment is built up based on the DeepMIMO data presented in Figure 2. The agent will interact with this simulated environment and learn from its experiences.
To train the model, we simulate this environment and evaluate the agent’s performance. This experience, consisting of the state and the reward, allows us to train our model by learning from its experiences. Repeating this process enough times leaves us with a model capable of controlling which micro base stations should be active or sleep-modus depending on the locations of its users, which can be deployed into the real world.
Conclusion
A key problem with 5G deployment is the technical complexity spanning across platforms and the cost to upgrade to 5G. With 6G on the horizon, optimising network coverage with ML to support these large volumes of data and high demand will become even more crucial.
By using Machine Learning, or more specifically Reinforcement Learning, we can optimise which base stations are active to provide optimal service and customer experience at lower costs for telecommunications companies. This solution further decreases costs by limiting excess energy consumption, resulting in significant value for both the business and society.
Datatonic is Google Cloud’s Machine Learning Partner of the Year with a wealth of experience developing and deploying impactful Machine Learning models and MLOps Platform builds. Our experience includes forecasting 5G usage with Vodafone and developing an MLOps platform for BT to reduce time to deployment from six months to six days. Need help developing an ML model, or deploying your Machine Learning models fast? Get in touch to discuss your requirements!