Ad Spend Optimisaton with Machine Learning: Your Questions Answered!
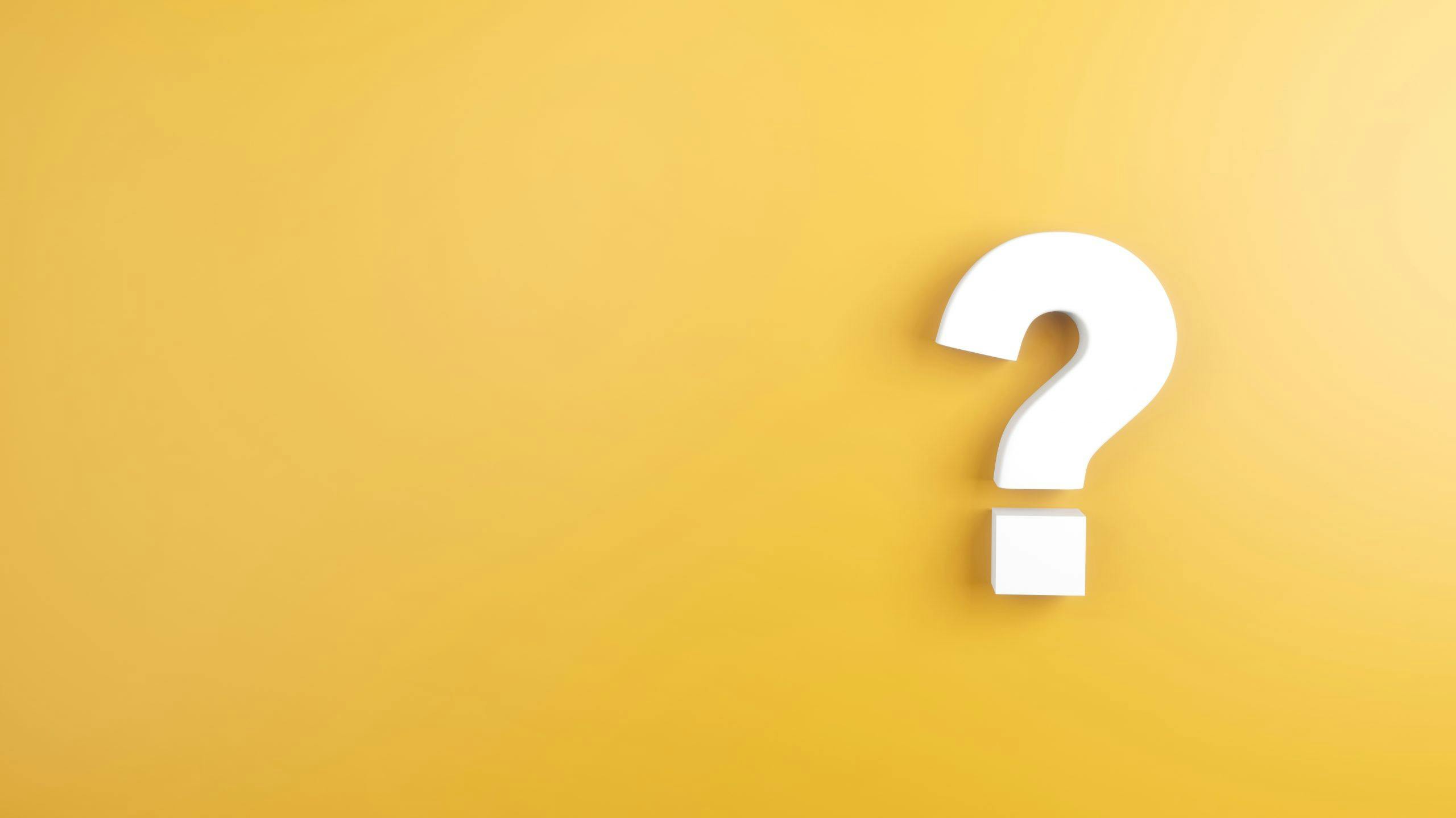
At our Ad Spend Optimisation event in September, we heard from Google Cloud’s Head of Digital Natives Nordics, Maria Wiss, about the importance of maximising the value from your first-party data as privacy controls increase, and our team covered two machine-learning driven strategies in the marketing analytics toolkit: Propensity Modelling and Marketing Mix Modeling.

Our attendees engaged our speakers, Avantika and Maria with a number of interesting questions, from defining success to maximising the value from particular machine learning-driven use cases. We’ve gathered some of our poll insights and answers to your questions here.
We’ve also made the slides available for download here.
Explainable AI (XAI)
Question: Do you look at ML explainability, and are you using Shapley Values?
Datatonic: Yes, absolutely. You can find a couple of case studies on our website where we looked at ML explainability. For News UK, we built an explainable AI module for a recommender engine, and we were looking at why a certain customer/customer type was being recommended a specific article in The Sun using Shapley values. For the Store Segmentation project, we also used Shapley values to explain what makes certain stores better-performing than others. For more information, see our XAI video series here.
Measuring the Success of Marketing Analytics Solutions
Question: Have there been real-life comparisons between estimated returns compared to actual? If so, how accurate have they been?
Datatonic: We’ve run multiple comparisons between forecast and actuals, across different marketing analytics projects. Our personalisation solution for luxury retailer, Mulberry, for example saw:
- +37% click-through rates in personalised email campaign
- +110% ROAS on Facebook Ads

Beyond marketing analytics, our sales + demand forecasting project for a large retailer, we observed:
- +31% increase in sales volume
- + 64% uplift in order fill rate
Broken Customer Journeys
Question: How can we utilise the data from broken customer journeys?
Datatonic & Google Cloud: This is a great question. It’s important to note that the broken customer journey is a journey as well – and it is only a part of the larger customer journey puzzle. This is when you can really leverage first-party data, and use the historical data of the customer. A Single-View Customer Platform like we delivered for Pets at Home could be helpful here to curate accurate consumer insights to take action from. For example, if you can then identify the person as one who has purchased before, and your solution can recognise those as a high-value customers, the broken journey is still valuable insight and can be used to reconnect with the customer via marketing campaigns for example. On the other hand, one of the important tasks is to identify the reason people leave a campaign or brand. You can use broken customer journey analysis, to identify whether it’s the product, the pricing, the messaging, or some other factor that makes the journey break. Once this is identified, you can use it to reach out to your customer and prevent the broken journey.
Marketing Mix Modelling
Question: Have you done MMM with any other attribution models than last click? e.g. behavioural attribution
Datatonic: We have used more traditional methods, such as last click attribution, as well as data driven attribution within the same project. We can work with different attribution models when forecasting such as behavioural attribution, and behavioural data can become part of advanced attribution models.

Would you like to know more details on our case studies and how we could achieve similar results for your business? Reach out to our team.