Data Mesh: Your Questions Answered
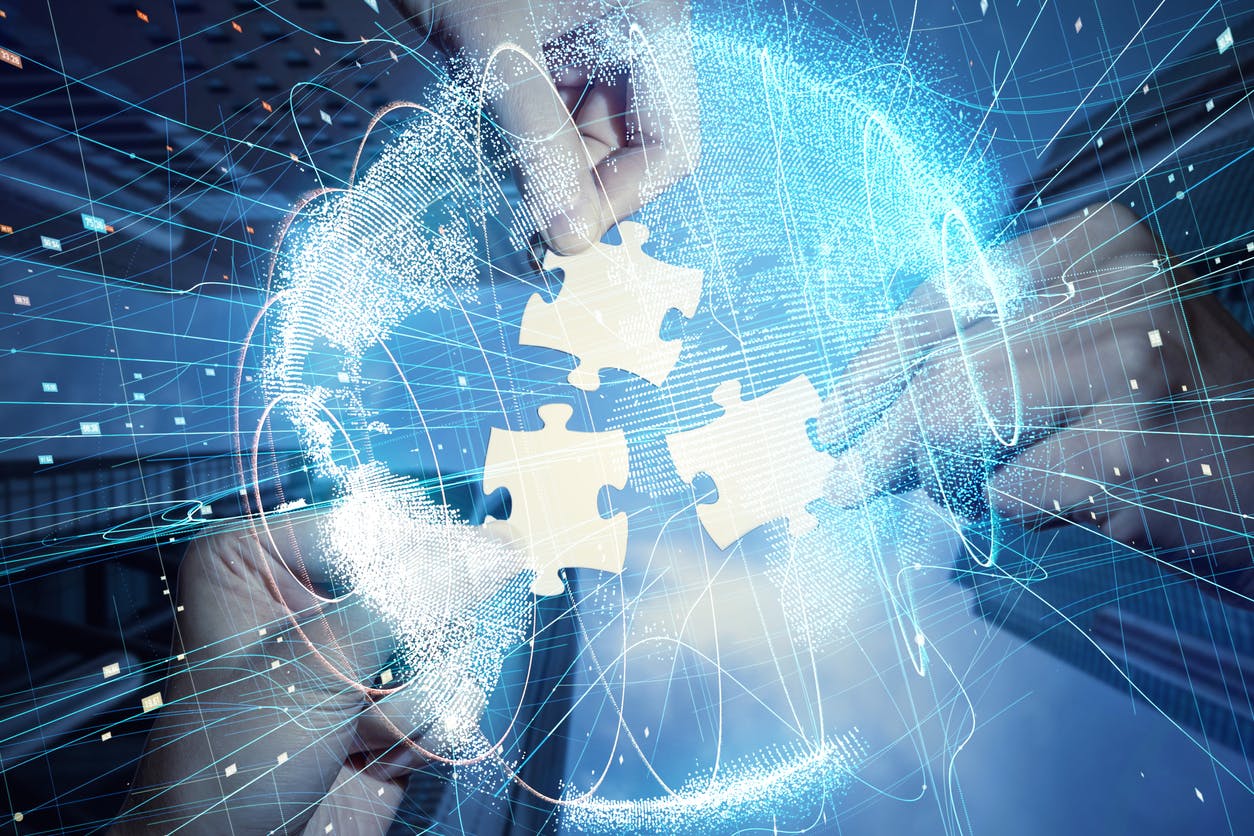

At our Data Mesh: From Principles to Practice webinar, Datatonic had the pleasure of welcoming data experts, Richard Oastler, Head of Data Engineering at Dojo, Jo Page, Director of Data and Data Science at OVO, and Bipul Kumar, Head of Data and AI Practice, Google Cloud.
Along with Datatonic’s Lead Cloud Architect, Andrew Harding, they discussed turning the concept of Data Mesh into reality.
We’ve seen for many years that organisations need data; they use it to make better business decisions, feed ML models, make predictions, and innovate. Historically, we have met this demand with technology, and have seen it evolve through various centralised solutions to collect data across the enterprise and serve it to the business.
Introducing Data Mesh
While we have seen lots of evolution in the technology space, the mindset of centralised data teams has remained the same. Data Mesh is an analytical data architecture and operating model where data is treated as a product and owned by teams that most intimately know and consume the data.
Data Mesh proposes a decentralised approach where individual departments build their own data capability within structured data domains. Each domain is responsible for building reusable and shareable high-quality data assets called data products. Domain teams apply their own expertise when building the products to benefit the entire organisation.
The following four principles work together for the successful implementation of Data Mesh:
- Data as a product
- Domain ownership
- Self-service data platform
- Federated computational governance
Combined, these principles eliminate the issues brought about by other data structures, such as data silos and inconsistency.
During our webinar, the audience gave us great insights into some of the common questions businesses might have when starting their Data Mesh journeys, ranging from the skills required for successful implementation to the challenges of data lineage and quality. Have a look at what our Data Leaders had to say.
Q + A: Your Questions Answered
1. Google is always staying one step ahead of the curve concerning emerging trends in Data and AI. How is Google approaching the Data Mesh hype and what are some of the biggest challenges on the radar that we’ll see as companies transition?
Google: Data Mesh is a transformational concept and it’s challenging the status quo by attempting to solve a key challenge which has been around for years. The challenge is to improve the agility around the usage of data and hence increase or maximise the value of data.
On the technology side, many of the building blocks are there, but the maturity needs to improve. The tooling is still evolving, especially in supporting newer concepts like federated centralised governance. Elsewhere, if you look at the organisational setup, many organisations don’t have the roles and personnel required for effective Data Mesh implementation. For example, finding a data product owner for every domain in the organisation who is considering Data Mesh is difficult.
It will take some time for it to become a common practice but as the toolchain matures and the skill set improves we will see more success stories and the perception will change.
“Tooling maturity and organisational change are super important when it comes to getting started in Data Mesh.” – Andrew Harding, Lead Cloud Architect, Datatonic
2. While Data Mesh is starting to develop as an evolution from centralised data platforms, users are asking whether there is still a place for a traditional Data Warehouse or whether Data Mesh acts as a replacement. Do you still see a need for a Data Warehouse?
Data Mesh offers a decentralised approach, but many companies are finding that this does not mean getting rid of their existing Data Warehouse, and are instead opting for a hybrid approach.
OVO: For us, there is still a need for a central Data Warehouse. With some data, it’s important that it is centrally owned, managed, and curated by a central data team.
However, the breadth of data in the warehouse must be monitored to make sure that businesses don’t just duplicate what is in the domains because that defeats the point of the Data Mesh. We are also working on making sure that data is easily-discoverable and interoperable across domains and this should diminish the need for how much data is in the warehouse.
Dojo: It’s important to highlight that moving to a Data Mesh does not mean ripping up everything that you’ve already put in place. We very much adopted the hybrid approach here at Dojo. With regards to challenges with maturity, we hope that products like Analytics Hub will simplify that and we can allow it to become much more isolated in the future but for us right now, that single project outputting your product from each domain is working well.
3. There will be several companies that have transitioned incrementally over the years from on-premise infrastructure to centralised solutions such as a Data Warehouse, that will already have somewhat more advanced data capabilities. What advice would you give to businesses looking to migrate to Data Mesh from legacy architecture?
There are many companies at the forefront of data for which a Data Mesh is just one step further on their data journey. However, this does not mean that Data Mesh is inaccessible for companies still operating with legacy on-premise infrastructure. By implementing Data Mesh in stages and prioritising the biggest issues, it does not need to be an overwhelming process.
Google: With Data Mesh, there are four principles, and you don’t have to start by implementing all four. If a business is migrating from legacy data architecture, it should identify its main pain point and then identify which one of those four or maybe more than one of those four can solve it. For example, if the biggest pain point is agility and we identify that our infrastructure is not flexible, then tackling the self-serve infrastructure is a good place to start.
The advice I would give is to pick one of those four pillars which will solve your biggest problem and then identify one or two use cases, and then think about building a Data Mesh from there. There will be a lot of learning depending on the organisation, but prioritising issues to solve will help businesses migrate efficiently.
4. How do you address skills gaps within domain teams and what can you do if teams aren’t starting from a very technical background?
The biggest point to understand is the niche differences between roles. It is important to know exactly what you are asking of each role and ensure that your teams understand this too. Understanding the skills and targets of each role will help to build balanced and capable teams.
OVO: The Data Mesh principles assume that you have the required skills. We’ve been on a journey to deeply understand roles such as Software Engineer and Data Engineer and look at how the two might work together. Businesses need to make it very clear what their expectations are of each role to fill those skills gaps.
Dojo: Different roles each add different skill sets to squads. If you know what each role is capable of, you can address areas where some aspects of data are not fully understood by forming multi-disciplinary teams within those domains as part of your strategy. You need to be flexible and understand what the skill requirements are for each area and resource it in that way.
5. Data lineage gives greater visibility while simplifying the ability to trace errors back to the root cause in data analytics processes. With Data Mesh, the domain data teams become responsible for the quality and usability of data. So, how can businesses approach the problem of data lineage with Data Mesh?
Dojo: We struggled with this in the early stages because the tooling is limited. We took two strategies on the transformation side.
Firstly, we built our own service to help with data transformation. This is difficult because there’s inconsistent naming between domains, standards, and approaches to how your presentation layer is constructed. We’ve got to the point now where we have an MVP that’s running as part of our CI/CD process that does that final check before things are merged into production.
Secondly, the other approach is a people-based approach where we’ve been very clear with our analysts about what the requirements are and what can break the Data Mesh. In the long run, we want to remove as much of that tacit knowledge as possible and put it into the tooling so no one needs to think about it. We would be able to operate in the same manner as if we had a large centralised repository as we did at the start.
6. Businesses have expressed some curiosity about Master Data Management (MDM) when using a decentralised approach to storing data. What are your thoughts regarding Master Data Management concerning Data Mesh? For example, is duplicate data okay?
Google: Master data management is important whether it’s in a Data Warehouse, Data Lake, or a newer Data Mesh. We can immediately see some challenges with MDM in Data Mesh because, by nature, it’s a central piece of information. Is it specific to a domain, should it remain central, or should it become its own domain? This will depend upon the organisation, but, more generally, the focus should be on providing and governing access to master data or reference data without duplicating it across every domain.
7. While separating data by different domains sounds useful in theory, how would you approach a scenario where you’ve got a use case that comprises multiple domains or products?
Dojo: It’s really important during the early stages of rolling out Data Mesh that you define your domains in a very clear way with the rationale behind them. We’ve been through several evolutions and if you’re getting to the point where you cannot put something in one of those domains, you’ve probably done it wrong. We’ve had to re-architect several times to counteract that but it’s a learning journey. Ultimately by design, they should be able to function in isolation with limited restrictions from those other domains.
8. With companies storing their data throughout the organisation, users are starting to ask whether a product catalogue is a key enabler for discoverability. To make products more discoverable, do the domain teams need to embrace some form of product catalogue?
OVO: I certainly resonate with the need for a data catalogue which would have data products within it. We found you can scale, particularly in your Machine Learning data products, those benefits by exposing them across multiple domains. This is where the need arose for the customer intelligence hub. If you build a Machine Learning data product within one domain, you can very quickly scale that by just exposing it through other domains. For that to happen, you need to know that a data product exists which brings the need for a catalogue and, very importantly, a common language to categorise other products.
Summary
To be successful in Data Mesh, you need to be holistic in your approach by understanding it from the perspective of people, processes, and technology. When implemented effectively, Data Mesh enables best-in-class data management capabilities.
There are new organisational structures with the creation of these domains and therefore new roles and skills will be needed within those domains to build out data capabilities. Tooling and maturity are also important. We need to let some tools mature and, in the short term, businesses may benefit from building some of their own capabilities to address those shortcomings.
“It will take some time for it to become a common practice but as the toolchain matures and the skill set improves we will see more success stories and the perception [of Data Mesh] will change.” – Bipul Kumar, Head of Data and AI Practice, Google Cloud UKI
Getting Started
If you’re considering the Data Mesh for your business, there are a few key considerations to help you get started.
- Understand where your organisation is currently.
A recurring theme from our discussions was the need to understand where your organisation is at the moment, before trying to start Data Mesh. This will inform your starting point, and ensure you have the right level of planning and understanding.
- Apply the principle that suits your business needs first.
Once you understand your current paint points, a solid next step is to apply just one or some of the Data Mesh principles such as thinking of data as a product. In other cases, starting to create that self-service data platform might be a very logical first step. However, we must note that in the long-run, Data Mesh functions best when all four principles are applied.
- If Data Mesh seems too abstract, start with a Data Mesh MVP.
Businesses should start with an MVP if they are struggling with the abstract nature of Data Mesh. For example, build a single domain or a single use case to start making some of the principles of Data Mesh more real to your organisation and foster some buy-in and sponsorship for such a large transformational program.
The key thing is to reflect on where you are in your organisation today, think about your strategy, and come up with an approach that works for you.
Need help deciding if Data Mesh could work for you? Get in touch to find out more.