MLOps in 2023
In this article, Datatonic’s Director of Machine Learning, Jamie Curtis, tells you how this year’s trends have answered key MLOps challenges and shares our top MLOps predictions for 2023.
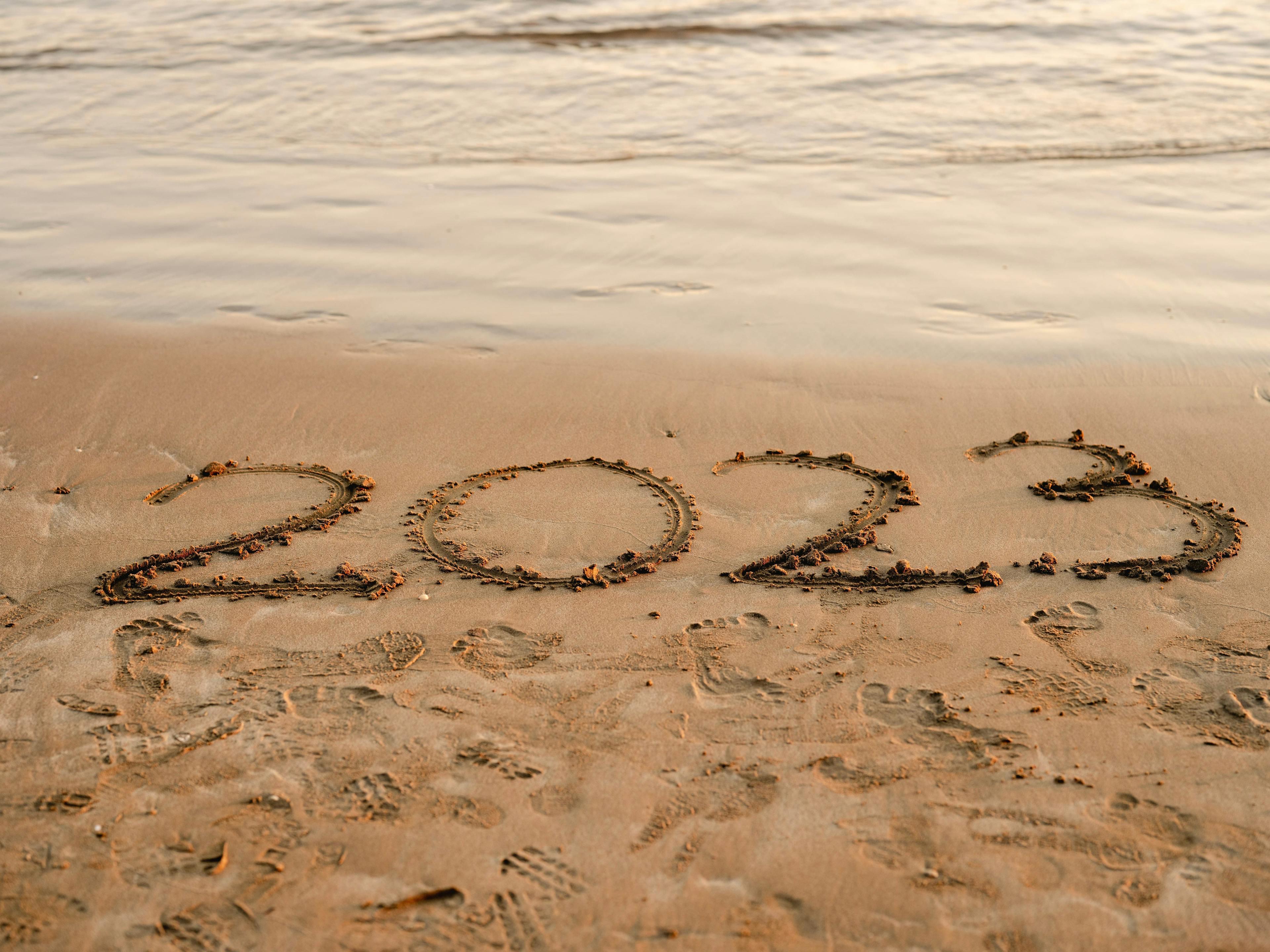
MLOps is a set of best practices, guidelines and technologies designed to deploy and maintain ML models in production. According to a recent McKinsey and Company report, MLOps can shorten the production time frame for ML applications by 90% and greatly reduce the required development resources.
This year, Datatonic has worked on MLOps projects for clients such as BT, Vodafone and Delivery Hero and experienced some of the biggest trends and challenges for companies looking to implement MLOps at scale, including data-centricity, real-time ML, and addressing skills gaps.
In this article, Datatonic’s Director of MLOps, Jamie Curtis, tells you how this year’s trends have answered key MLOps challenges and shares our top MLOps predictions for 2023.
The Rise of Centralised MLOps Platforms
Some companies, especially large ones, are realising that they need centralised fundamental MLOps platforms.
“This year, we developed large-scale centralised end-to-end MLOps platforms for clients such as Vodafone and BT to enable them to scale Data Science across the business, and automate as much of the ML lifecycle as possible. This includes automation of deployment processes, training and retraining, serving, and monitoring; they want to be able to automate everything.” – says Jamie Curtis, MLOps Practice Lead, Datatonic
A primary driver for this is to serve Data Scientists as it allows them to focus on Data Science and automate processes which are inhibitive to that, like networking, security controls, access permissions, infrastructure management, and data engineering.
Companies are Rapidly Building MLOps Skillsets
In 2022, we’ve seen a large realisation of the need for skillsets and roles for people that can work within ML. Companies are realising that they need unique skill sets they don’t yet have, like those of ML Engineers, to achieve their business goals.
According to O’Reilly’s 2022 State of AI Adoption in the Enterprise, only 26% of enterprise organizations have models in production, with respondents blaming lack of key skills as the biggest barrier to deployment.This gap between required skills and available talent is one of the reasons that Datatonic Academy was built. By providing customised instructor-led training courses, Datatonic Academy has successfully supported some of our largest customers to upskill their teams.
Significant MLOps Trends in 2023
Based on a wealth of experience within MLOps, delivery projects for companies such as BT, Vodafone and Delivery Hero, Datatonic has solved common challenges for companies looking to implement MLOps. After noticing the most significant trends and challenges for MLOps in 2022, here are our top three predictions for 2023.
- Increased focus on Data-Centricity
Data-Centric AI is shifting the focus from a model-centric to a data-centric approach in AI. Because an ML model is only as reliable as the data used in it, improving the quality of data improves outcomes. To maximise the value of their ML, businesses need to implement best-in-class data practices. However, most data platforms don’t take their companies’ data to a level of maturity advanced enough for their ML platforms.
This leaves a gap where extra effort is required to process the data before the ML models can be trained efficiently.
There are two main ways to address this gap:
- Developing data engineering capability by extending the reach of end data products
- Developing data preprocessing capabilities by providing more scalable or sophisticated methods for data cleansing, data validation, data splitting and feature engineering
Solving this gap will be a significant area of focus moving forward to simplify the process of implementing MLOps.
- Reducing Entry Barriers for Data Scientists
Companies are already starting to make investments in reducing entry barriers for Data Scientists as much as possible. For Delivery Hero, we created a centralised MLOps platform to meet the needs of Data Scientists across the business with a focus on increased automation and reduced entry barriers for Data Scientists. This enabled them to experiment with ML models and then deploy those models much more easily and quickly.
We’ve noticed that there is quite a significant initial investment in upskilling that Data Scientists need to commit to before they can engage effectively with the MLOps platform that enables them to focus on Data Science. When scaling a platform across a large company, it’s optimistic to assume that everyone will commit to this learning curve and meet the required skill levels. Tools such as Command Line Interfaces (CLIs) can significantly reduce the amount of time and money spent on upskilling large teams, especially when used at scale.
- More Real-Time ML
MLOps will also enable more real-time ML. As MLOps becomes more advanced, more companies will look to implement real-time ML. This is ML that learns in real time and constantly improves based on the data that it receives. Over time, this will enable models to become more accurate than ever before with less manual training required.
The MLOps infrastructure that enables real-time ML is becoming more common and more accessible. With MLOps becoming easier for Data Scientists, many more companies will seek to implement real-time ML for a range of applications.
All of This is Possible with Google’s Vertex AI…
Vertex AI offers a great range of solutions and a great depth of solutions. It provides complex aspects of MLOps as well as low-code and no-code options like AutoML and pre-trained APIs that are based on Google’s open datasets which are a lot more accessible. Other more niche tools and services only offer either breadth or depth, but not both.
It also integrates well with other Google Cloud products such as the NLP API. Google focuses a lot on auxiliary and forward-thinking solutions such as explainable AI and responsible AI. They’re things that aren’t necessarily widely used or in demand right now, but they will be in the near future.
Vertex AI benefits directly from and builds upon, Google’s world-leading research into state-of-the-art Machine Learning. It’s grounded in Google’s commitment to open source, providing greater portability, security, and reduced vendor lock-in.
Finally, it’s fully modular; you can use Vertex AI’s built-in solutions, but you can also build customised capabilities on top of Vertex AI using best-in-class technologies from open-source communities and partners (such as NVIDIA, Labelbox, etc.). As organisations are increasingly relying on their AI/ML capability for competitive advantage, the need for flexible and innovative ML platforms is greater than ever before.
Conclusion
MLOps is a practice with several clear advantages for businesses looking to generate real business value.
Over the next year, we expect to see the following:
- An increased focus on data quality
- Lower entry barriers for Data Scientists
- More real-time ML
In 2023, MLOps will only become even more powerful in pushing the boundaries of ML and Data Science forward.
Datatonic is Google Cloud’s Machine Learning Partner of the Year with a wealth of experience developing and deploying impactful Machine Learning models and MLOps Platform builds. Need help developing an ML model, or deploying your Machine Learning models fast?
Have a look at our MLOps 101 webinar, where our experts talk you through how to get started with Machine Learning at scale or get in touch to discuss your ML or MLOps requirements!