Understanding Your Data Stack Personnel and Tooling Costs
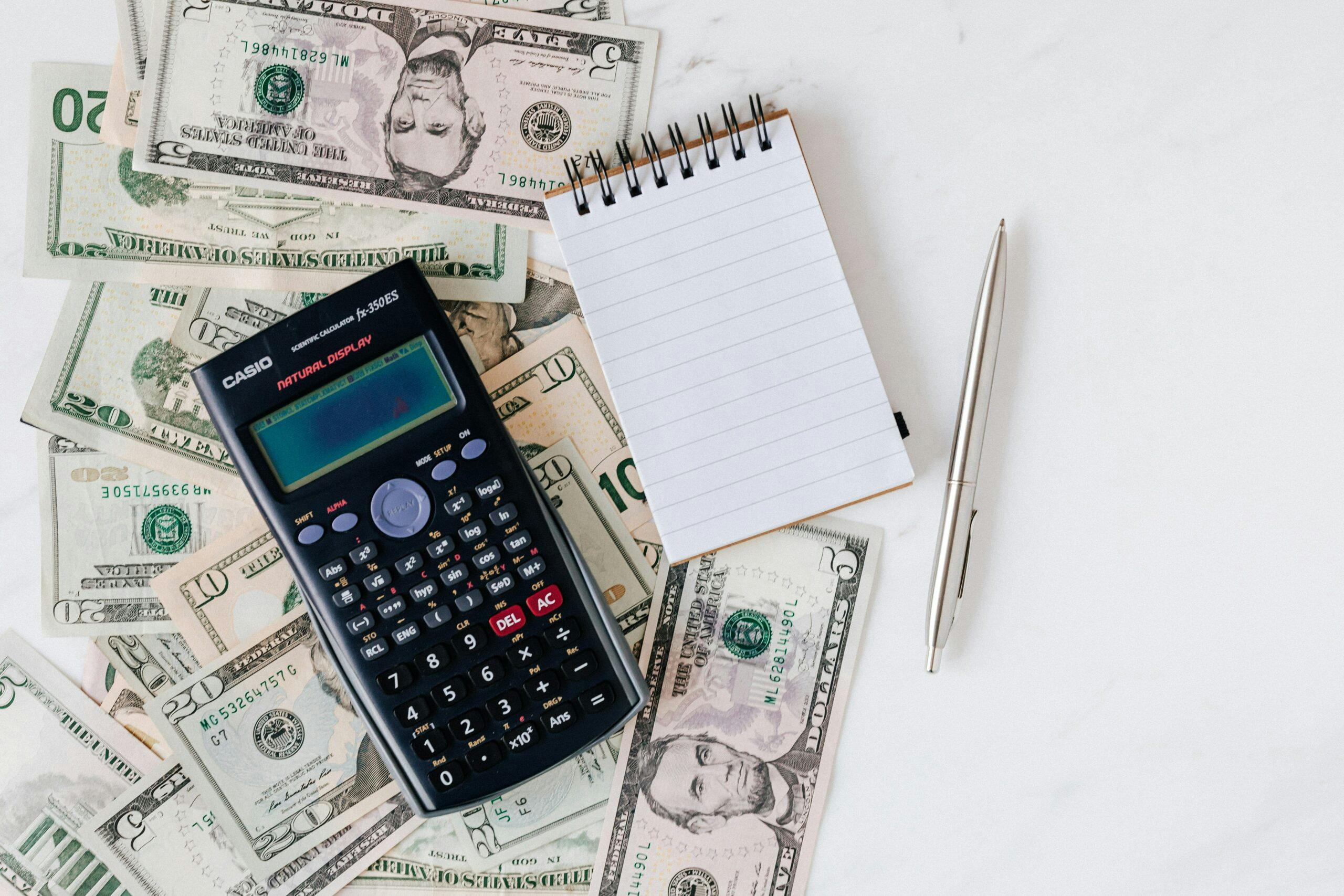
Author: Yoni Hazan, Lead Analytics Consultant
When it comes to calculating the costs associated with a data team, the task is complex as there are several different factors that impact it from a financial perspective. Two major cost categories to consider in this calculation are personnel costs and the cost of data tooling. Understanding how to calculate these costs, and the return on investment associated with them, is critical to building an effective data stack.
In this article, we discuss these two areas, the key factors to consider when calculating the costs associated with both, and how investing in personnel and data tooling can position your business for long-term, scalable growth and success.
Personnel Cost
The cost of a data team is the most important cost within your department as the team generates value regardless of the data tools used. The size of the team depends on the needs and size of the business. Below, is a general estimate of how data teams should be structured in relation to the size of the overall organisation.
Small business (10–50)
Your data team should be representative of one or two team members where the roles and responsibilities are divided between development, gathering requirements and prioritisation of the most valuable items that will help move the needle. These team members need to be flexible and adaptable to the changing needs of the team, working across architecture, data ingestion, modelling, and analysis.
Medium business (50–200)
Generally, medium-sized businesses will want to have a team of three to five members of which the roles of each team member will be more defined. Here’s an example team composition for an organisation of that size, with head counts depending on your data and business needs:
- One Team Lead or Manager directing the team, communicating with leadership across the organisation, and managing all individual team members.
- One Product Owner responsible for refining work and being responsible for ensuring that proposed work produces business value.
- One Data Engineer responsible for integrating data into a centralised data warehouse. More data engineers may be needed if there are a lot of complex data sources and fewer or none may be needed if third-party ingestion is sufficient (e.g., with Fivetran).
- One Analytics Engineer responsible for translating source-centric data into a business-centric data model that is ready for analysis or use. More analytics engineers may be needed if the logic or data is complex.
- One Analyst responsible for analysing data and identifying actionable insights within that data. More analysts may be needed if there are more complex analysis needs and fewer or no analysts may be needed if other functions are already self-serving their own analysis (e.g., Marketing could be sufficiently trained on the BI tool and able to handle their own analysis needs).
Large business (200+)
For larger businesses, the size of the team should exceed five people to support the main needs of the company. This is due to the amount of data sources, data needs, as well as the need to maintain a robust data stack that can support the business needs. In larger businesses, it is essential to have a team lead who can identify where ROI is impacted the most, a business analyst who can gather requirements and provide visuals, analytics engineers, and a data engineer.
Data Tooling Costs
Above, we recommended rough guidelines for roles and headcount for different stages of your organisation’s growth. With that in mind, you can think of your tooling cost as your second major cost category and one that often works hand-in-hand with personnel costs. Teams must identify the most essential technologies and tools necessary for day-to-day work, and expand from there.
Let’s explain that in more detail with some examples. With any data tools, the costs associated can be split by the upfront costs, and the ongoing costs of managing and maintaining platforms.
Upfront Costs
The upfront costs associated with tooling include initial software cost, servers and storage costs as well as implementation or migration costs, in the form of hiring a team of developers or consultants to set up and configure new tools.
When calculating the upfront costs of a tool, businesses should consider the following factors:
- The volume of data being stored and processed
- The complexity of the data, including the number of sources and data types
- The performance requirements for querying and processing data
- The data governance requirements, including security and compliance
Ongoing Costs
The ongoing costs of data tooling include the cost of maintenance, keeping licensing up to date and operational and the staffing required, either through retaining a data team, explained above, or in working with consultants.
When calculating the ongoing costs of data tooling, businesses should consider the following factors:
- The forecasted growth of data over time
- As volume and complexity grow, the performance requirements may impact the need for additional tooling to maintain performance and scale.
Potential Cost Savings
While the costs of these tools can be significant, there are potential cost savings that businesses can realise by implementing these tools, including:
- Increased efficiency – By centralising data storage and processing, businesses can increase efficiency and reduce the time and cost of data integration and transformation.
- Improved decision-making – By providing access to timely and accurate data, businesses can improve decision-making and reduce the cost of errors and missed opportunities.
- Scalability + Lower Maintenance Costs – Modern data tools can scale to handle increasing data volumes and complexity, reducing the need for additional investments.
- Automation + Time Saving – By automating data integration, transformation, and processing, businesses can reduce the cost of manual labour and increase productivity.
A common issue organisations face when evaluating a modern data stack is identifying which tools to invest in relation to their unique business needs. Tools can be segmented into two categories, core tools and satellite tools. Below, we give a breakdown of these tool categories and some examples of tools Montreal Analytics recommends to its clients.
Core tools
Without these core tools, the data team will likely have very limited capacity. In some cases, you’ll be able to get the work done on your own through custom ingestion or analysis with free tools like Google Sheets, but in general, these categories require some spending.
Automated Ingestion
Automated Ingestion tools can be seen as the first element in your modern data stack, and the first core tool to consider when evaluating implementation, and the costs associated with this. Ingestion tools synchronise data from various sources and applications to a destination, like your data warehouse, using pre-built connectors.
Data Warehousing
Data Warehousing tools, such as Snowflake or BigQuery, serve as a centralised repository for all of an organisation’s data being pulled in from disparate sources from which the power of business-generated data can be harnessed.
A business’ data warehouse will likely drive the majority of costs associated with your data tooling — impacted mainly by query time, as well as costs associated with running transformation jobs on a specific cadence to curate data and make it available for consumption to the end user.
BI Tooling
BI tools, such as Looker enable data teams to create reports and dashboards that can be used to visualise data, identify opportunities within it and share real-time business analytics.
Satellite Tools
These tools are not core to most business needs, but they can provide either a lot of productivity gains or time savings/personnel cost savings.
Reverse ETL
Tools like Census or Hightouch sync customer data from your warehouse or BI tools into CRM, email marketing, advertising tools and more to enable other teams and departments to act on real-time data.
Data Observability
Tools like Monte Carlo, Datafold or Metaplane enable data teams to monitor for missing or inaccurate data to ensure problems and data quality issues are identified and resolved in real-time.
Data Cataloguing
Data cataloguing tools like Atlan act as a single source of truth and information for teams and an inventory of all data assets in an organisation, helping data teams discover and understand appropriate data for analytical or business purposes.
Conclusion
Calculating the costs associated with personnel and data tooling in a modern data stack is a complex task. However, it is critical to understand how to calculate these costs and the return on investment associated with them. Personnel cost is the most important cost within a data team, and the size of the team depends on the needs and size of the business.
Data tooling costs also play a crucial role in the calculation of the overall cost. While representing a significant investment, by implementing modern data tools, businesses can realise potential cost savings such as increased efficiency, improved decision-making, scalability, and automation.
For more information on what you can do to manage costs and gain visibility over your spending, take a look at our FinOps whitepaper, 4 Steps to Cloud FinOps Maturity. Datatonic has a wealth of experience in data + AI. To discuss your challenges or work on a project together, contact us here.