Responsible AI 101: What You Need to Know
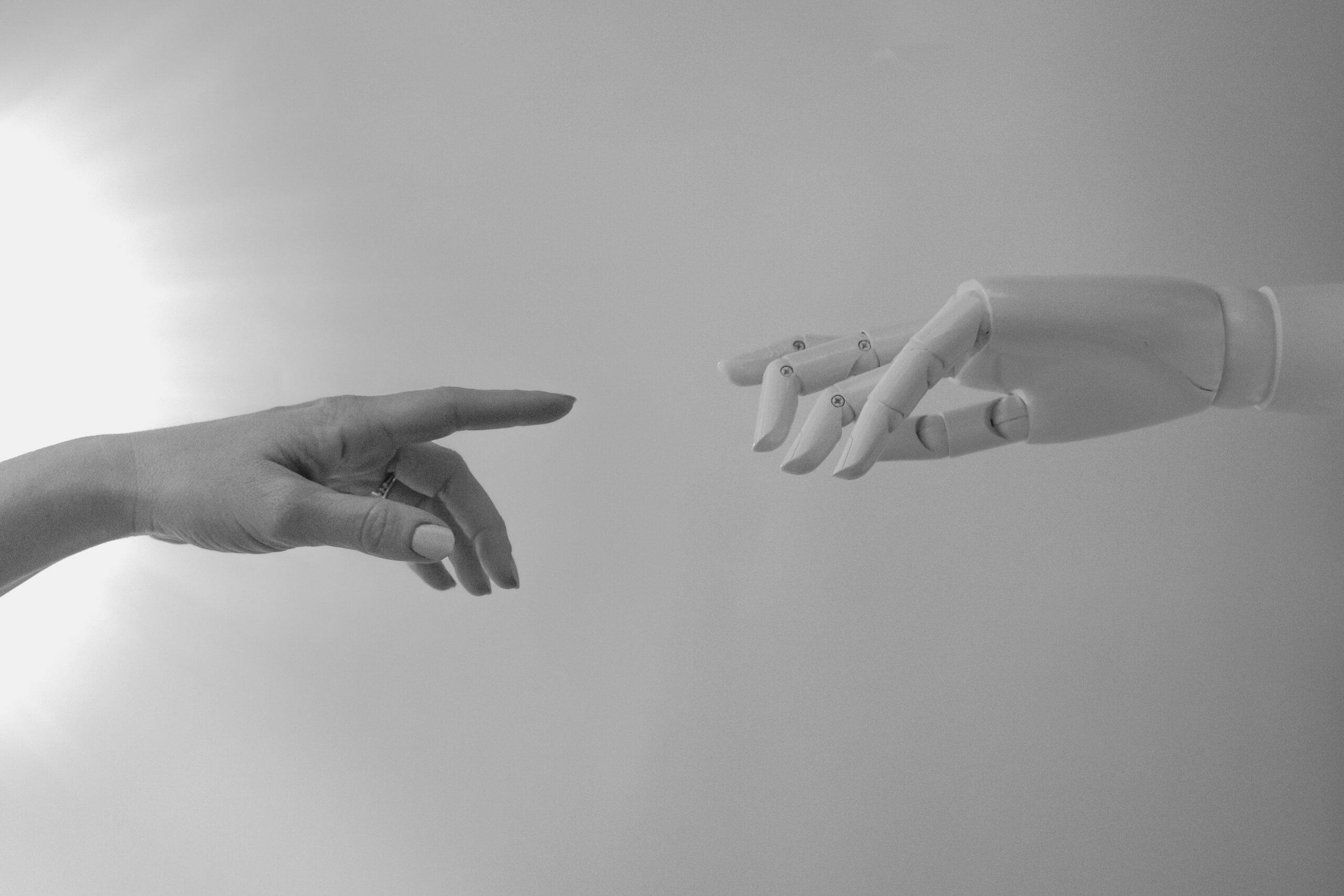
Authors: Valentin Cojocaru, Data Science Lead, Matthew Gela, Senior Data Scientist, Christelle Xu, Machine Learning Strategy Lead
AI and Machine Learning have rapidly evolved to become an integral part of our daily lives, and are being increasingly integrated into business processes to unlock value. With that, there is an increased necessity to ensure AI is developed equitably, securely, transparently and accurately. In particular, the recent wave of releases of models such as Bard and DALL-E to the public has undoubtedly brought an increased level of opportunity, but also an unprecedented spotlight on the use and misuse of AI.
The UK Government’s new whitepaper on AI Regulation illustrates the importance of integrating Responsible AI into your AI systems. In the age of Generative AI, Responsible AI is more important than ever, and it is crucial for businesses to understand what it actually is. In this first blog of our Responsible AI series, we’ll discuss the context for Responsible AI, why it matters and what it means to develop AI systems responsibly.
Why Responsible AI Matters
“Technology is neither good nor bad; nor is it neutral.” – Melvin Kranzberg
Our society has developed an unconscious trust in technology and engineering, largely focusing on the boundless upside of technological advancement, while at the same time trusting that these technologies are built safely and fairly; we don’t commute to work fearing our trains might suddenly crash. The same mindset has been applied in the field of AI, and over the last decade, many organisations have rushed to create and invest in Machine Learning teams that focus on delivering business value as quickly as possible, often misunderstanding the implications of ML systems that are built with outcomes, and not processes in mind. We don’t need to dig far to see examples of this, including some very high-profile ones.
The good news is that Machine Learning as both a topic and industry solution has become widely adopted, and now industries, governments and the public have started to understand the nuances and implications of building AI systems. Guidelines and principles have been made available by bodies such as the ICO, providing organisations with standards in which to develop AI systems.
Increasing Regulations
Governmental bodies have proposed, and in some cases already created, sweeping legislation to both control the misuse of AI and instil greater transparency in building and monitoring AI systems in production. Early preparation and adoption of the requirements of these policies should be incentivised and enforced sooner rather than later, especially for companies that have built a large number of ML applications in their portfolio and platforms. Understanding regulations, auditing their ML systems, creating blueprints and frameworks to create responsible ML applications by design and actively enforcing these practices takes time and effort.
For example, the EU is expected to pass the EU AI Act in 2023, constraining the use of ‘high-risk’ ML systems such as some of the systems used in areas such as infrastructure, policing, biometric surveillance, and recruitment. Another key aspect of the legislation is the inclusion of certain requirements when building and monitoring these systems, such as the use of high-quality data, documentation, traceability, transparency, human oversight, accuracy and robustness.
In the US, federal, state and municipal laws already touch on ML applications, such as the Equal Credit Opportunity Act and the Fair Housing Act at a federal level, while at the state level New York, Illinois and Maryland have passed laws to mandate bias audits for automated employment decision tools that use AI to assist in candidate screening. More broadly, the White House has released the Blueprint for an AI Bill of Rights, which provides guidance for companies and individuals to protect them from threats presented by AI. China has also passed a regulation that controls the use of algorithms in online recommendation systems.
Developing AI Systems Can be Risky
Outside of any potential legal implications of not adhering to laws and regulations, there are other, equally important reasons why a company should build and maintain responsible ML systems. Companies should consider the cost of a wrong outcome or decision in their business. Could the system have an impact on the customer lifetime value? Are there any other brand or reputational risks involved? Could the system produce deep harm to end users or the general public? Are there any operational risks involved?
Responsible AI is most critical in systems that involve or affect decisions that impact human well-being, including access to resources or their health. For a company, Responsible AI can act as a differentiator, particularly with customers who choose to do business with organisations that align with their values.
What Does it Mean to Develop AI Responsibly?
The emerging field of ML risk mitigation practices has been coined as Responsible AI. Responsible AI does not have a strict and standardised definition, and we should instead aim to define it as a set of principles that govern the responsible and ethical use of AI, in a way that fairly impacts customers and society as a whole. It is usually comprised of the following five pillars:
Bias + Fairness
Fairness in Machine Learning means ensuring that the decisions and predictions made by a model should not unfairly advantage or disadvantage any particular individual or group in society. All ML models show some level of bias, but significant bias, or bias within high-impact use cases, can cause legal liability or real-world consequences. Systemic bias and statistical bias can lead to differential outcomes and discrimination. This can have serious ramifications in domains such as criminal justice, hiring and lending.
One high-profile example of this is the criminal risk assessment algorithm (COMPAS) used by judges and parole officers to predict the likelihood of reoffending in some US states, which was found to give significantly higher risk scores to people from ethnic minority backgrounds. Since data is tightly coupled to the functionality of ML models, care needs to be taken when collecting and understanding data, as well as selecting features for modelling. Detecting, mitigating and reporting bias in ML models across the modelling pipeline (from checking for data integrity at the time of collection all the way to reporting model results against feature distributions) is crucial.
Explainability
Explainability in AI is the set of processes and methods that allows human users to understand and trust the results and output created by machine learning algorithms.
In order to determine whether your AI system is safe for making decisions or augmenting business processes, it is crucial to understand how it comes to a decision. Without this, you can have situations where the model could be basing its predictions on information it should not be relying on. Explainability enables developers to debug their models, stakeholders to understand whether the AI system is fit for purpose, and end users to qualify whether they can trust the model output.
Activities such as engineering meaningful features, generating explanations for individual model predictions, and setting up systems for tracking and creating thorough documentation of models are all ways to incorporate explainability into AI systems.
Sustainability
Sustainability in AI is the practice of developing and using machine learning models in a way that minimises their environmental impact and promotes long-term societal and economic sustainability. As the use of AI and machine learning grows rapidly, the environmental impact of these technologies becomes more significant.
By adopting sustainable practices, we can ensure that these technologies are used in a way that benefits both society and the planet. Measuring energy consumption allows you to determine whether your energy usage is high, and provides insight into areas where consumption could be reduced. In a previous blog, we looked at how to predict the energy consumption of machine learning models based on the number of CPU operations they require.
Other methods to become more sustainable include reducing the amount of data required for training and deploying models, using models that require fewer resources to train and use, as well as optimising hardware usage by using compute resources more efficiently.
Robustness
Robustness ensures that machine learning systems can be monitored and perform reliably in different scenarios and over time. A robust AI system should be able to generalise well to new or unseen data and be resistant to minor changes in the data distribution. In many domains, such as healthcare and autonomous vehicles, the reliability of machine learning models is crucial. A model that is not robust may make incorrect decisions even when the input data is only slightly different from the training data, leading to undesirable consequences.
To improve robustness, you can set up monitoring and alerting designed to perform checks such as detecting data drift and sending an alert when the drift is high. Additionally, validating that the input data to a model is good quality allows the model to train and predict on clean and consistent datasets, which is a crucial step in improving the robustness of a model. In our Vertex AI Tips & Tricks series, we have discussed how you can implement data validation in your machine learning pipelines using Great Expectations, and how you can set up alerting with Google Cloud Monitoring.
Privacy + Security
Privacy and security in AI refers to the protection of sensitive and confidential data used by machine learning models, and ensuring that the models are secure against adversarial attacks.
Taking privacy-preserving measures is crucial for protecting the personal information that organisations store about individuals, preventing it from being misused or shared without consent. Fortunately, there are techniques that can be implemented to enhance privacy, such as data anonymisation, using privacy-preserving machine learning algorithms, or using a federated learning strategy to allow models to be trained across multiple devices or servers without exchanging data.
Model security prevents unauthorised access to machine learning models, protecting them from theft, modification or misuse. Models may also be susceptible to adversarial attacks, which is where attackers can generate examples that are designed to deceive the model into making incorrect predictions, leading to undesirable consequences. Techniques such as adversarial training and defensive distillation can improve the model’s resistance to adversarial attacks.
Conclusion
As AI becomes more widespread, it is crucial that we consider all five key pillars of Responsible AI. In the upcoming blogs in this series, we will expand on how your development teams can incorporate these pillars within your machine learning workflows, and we will demonstrate how to implement and monitor these within a production pipeline. We’ll also look at the wider picture, and give our take on why we believe Responsible AI is crucial in shaping the story of Generative AI towards something beneficial for society.
P.S. Although very tempting, this blog was not written by generative AI…
Datatonic is Google Cloud’s Machine Learning Partner of the Year with a wealth of experience developing and deploying impactful Machine Learning models and MLOps Platform builds. Need help developing an ML model, or deploying your Machine Learning models fast?
Have a look at our MLOps 101 webinar, where our experts talk you through how to get started with Machine Learning at scale or get in touch to discuss your ML or MLOps requirements!